Adding GPUs to Applications
Service
, Job
, Model
and Notebook
applications can now easily use one or more Nvidia GPUs for accelerated machine learning training and inference.
Availability
Note
- Currently only one or more full GPU units can be alloted to any application. Please reach out to us if you have use cases that can benefit from smaller units of a single GPU unit.
- Currently we only support NVIDIA GPUs, although special acclerators like AWS Graviton, AWS Traniuim, AWS Inferentia, Google TPUs and AMD GPUs can be provisioned and used with additional effort. Please reach out to us if you have use cases that benefit from these accelerators.
We currently support the following GPUs on the Truefoundry Platform. Each cell denotes the count of GPUs that can be requested for that type of GPU unit.
Type | AWS EKS | GCP GKE Standard | GCP GKE Autopilot | Azure AKS |
---|---|---|---|---|
K80 (12 GB) | Â Up to 16 | Up to 8 (Retiring May 1, 2024) | NA | Retired |
P4 (8 GB) | NA | Up to 4 | NA | NA |
P40 (24 GB) | NA | NA | NA | Retired |
P100 (16 GB) | NA | Up to 4 | NA | Retired |
V100 (16 GB) | Up to 8 | Up to 8 | NA | Based on the Nodepool Instance Size |
T4 (16 GB) | Up to 8 | Up to 4 | 1, 2, 4 | Based on the Nodepool Instance Size |
A10G (24 GB) | Up to 8 | NA | NA | Based on the Nodepool Instance Size |
A100_40GB (40 GB) | Up to 8 | Up to 16 | 1, 2, 4, 8, 16 | Based on the Nodepool Instance Size |
A100_80GB (80 GB) | Up to 8 | Based on the Nodepool Instance Size | NA | Based on the Nodepool Instance Size |
L4 (24 GB) | NA | Based on the Nodepool Instance Size | NA | NA |
H100 (80 GB) | On Roadmap | On Roadmap | NA | On Roadmap |
Based on the Nodepool Instance Size
means 1 to max number of GPUs available per node in the Nodepool.
Please note that GPU availability is subject to your cloud provider quotas and region limitations applied to your respective cloud accounts.
Compatibility Notes
L4
GPUs on GKE need GKE v1.25 or higher with Container Optimised OS Version 101 (minimumcos-101-17162-127-8
) or higherA100_80GB
andL4
on GKE cannot be used using Node Auto Provisioner.
Generative AI Examples
Adding GPU to Service
, Job
or ModelDeployment
Service
, Job
or ModelDeployment
A GPU can be easily attached by passing in gpu_count
and servicefoundry.NodeSelector
/ servicefoundry.NodePoolSelector
to Resources
part of the Service
, Job
or ModelDeployment
definition like the following:
Using Auto Provisioning
Azure and Generic clusters do not support Auto provisoning, please use Nodepools method
import logging
from servicefoundry import Build, Port, PythonBuild, Resources, Service, LocalSource
+ from servicefoundry import NodeSelector, GPUType
logging.basicConfig(level=logging.INFO, format=logging.BASIC_FORMAT)
service = Service(
name="stable-diffusion-v21",
image=Build(
build_spec=PythonBuild(
python_version="3.8",
requirements_path="requirements.txt",
command="python app.py"
),
),
ports=[Port(port=8080, expose=False)],
resources=Resources(
cpu_request=3.5,
cpu_limit=3.5,
memory_request=14000,
memory_limit=14000,
ephemeral_storage_request=50000,
ephemeral_storage_limit=50000,
+ gpu_count=1,
+ node=NodeSelector(gpu_type=GPUType.T4)
)
)
service.deploy(workspace_fqn="...", wait=False)
name: stable-diffusion-v21
type: service
image:
type: build
build_spec:
type: tfy-python-buildpack
command: python app.py
python_version: '3.8'
requirements_path: requirements.txt
build_context_path: ./
build_source:
type: local
ports:
- port: 8080
expose: false
protocol: TCP
replicas: 1
resources:
cpu_limit: 3.5
cpu_request: 3.5
memory_limit: 14000
memory_request: 14000
ephemeral_storage_limit: 50000
ephemeral_storage_request: 50000
+ gpu_count: 1
+ node:
+ type: node_selector
+ gpu_type: T4
Using Nodepools
For this, please first register your already created nodepools in Integrations > Clusters > Edit Cluster
GKE Autopilot does not support creating Nodepools, please use Auto Provisioning method
import logging
from servicefoundry import Build, Port, PythonBuild, Resources, Service, LocalSource
+ from servicefoundry import NodepoolSelector
logging.basicConfig(level=logging.INFO, format=logging.BASIC_FORMAT)
service = Service(
name="stable-diffusion-v21",
image=Build(
build_spec=PythonBuild(
python_version="3.8",
requirements_path="requirements.txt",
command="python app.py"
),
),
ports=[Port(port=8080, expose=False)],
resources=Resources(
cpu_request=3.5,
cpu_limit=3.5,
memory_request=14000,
memory_limit=14000,
ephemeral_storage_request=50000,
ephemeral_storage_limit=50000,
+ gpu_count=1,
+ node=NodepoolSelector(nodepools=["my-gpu-nodepool"])
)
)
service.deploy(workspace_fqn="...", wait=False)
name: stable-diffusion-v21
type: service
image:
type: build
build_spec:
type: tfy-python-buildpack
command: python app.py
python_version: '3.8'
requirements_path: requirements.txt
build_context_path: ./
build_source:
type: local
ports:
- port: 8080
expose: false
protocol: TCP
replicas: 1
resources:
cpu_limit: 3.5
cpu_request: 3.5
memory_limit: 14500
memory_request: 14500
ephemeral_storage_limit: 50000
ephemeral_storage_request: 50000
+ gpu_count: 1
+ node:
+ type: nodepool_selector
+ nodepools:
+ - my-gpu-nodepool
Please see the above table for supported GPU types on respective cloud providers and cluster types.
Adding CUDA Toolkit
Additionally, your application might want to have CUDA toolkit installed. If you are using PythonBuild
, you can configure it simply by passing it as cuda_version
import logging
from servicefoundry import Build, Port, PythonBuild, Resources, Service, LocalSource
+ from servicefoundry import NodeSelector, NodepoolSelector, GPUType
+ from servicefoundry import CUDAVersion
logging.basicConfig(level=logging.INFO, format=logging.BASIC_FORMAT)
service = Service(
name="stable-diffusion-v21",
image=Build(
build_spec=PythonBuild(
python_version="3.8",
+ cuda_version=CUDAVersion.CUDA_11_3_CUDNN8
requirements_path="requirements.txt",
command="python app.py"
),
),
ports=[Port(port=8080, expose=False)],
resources=Resources(
cpu_request=3.5,
cpu_limit=3.5,
memory_request=14500,
memory_limit=14500,
ephemeral_storage_request=50000,
ephemeral_storage_limit=50000,
+ gpu_count=1
+ node=NodeSelector(type=GPUType.T4),
# or using nodepool:
# node=NodepoolSelector(nodepools=["my-gpu-nodepool"])
)
)
service.deploy(workspace_fqn="...", wait=False)
name: stable-diffusion-v21
type: service
image:
type: build
build_spec:
type: tfy-python-buildpack
command: python app.py
python_version: '3.8'
requirements_path: requirements.txt
build_context_path: ./
+ cuda_version: 11.3-cudnn8
build_source:
type: local
ports:
- port: 8080
expose: false
protocol: TCP
replicas: 1
resources:
cpu_limit: 3.5
cpu_request: 3.5
memory_limit: 14500
memory_request: 14500
ephemeral_storage_limit: 50000
ephemeral_storage_request: 50000
+ gpu_count: 1
+ node:
+ type: node_selector
+ gpu_type: T4
# or using nodepools
# node:
# type: nodepool_selector
# nodepools:
# - my-gpu-nodepool
Check servicefoundry.CUDAVersion
enum for all available CUDA versions and cuDNN versions.
Alternatively, you can bring your own docker image or Dockerfile which has CUDA Toolkit pre-installed.
Configuring GPU and CUDA Version from UI
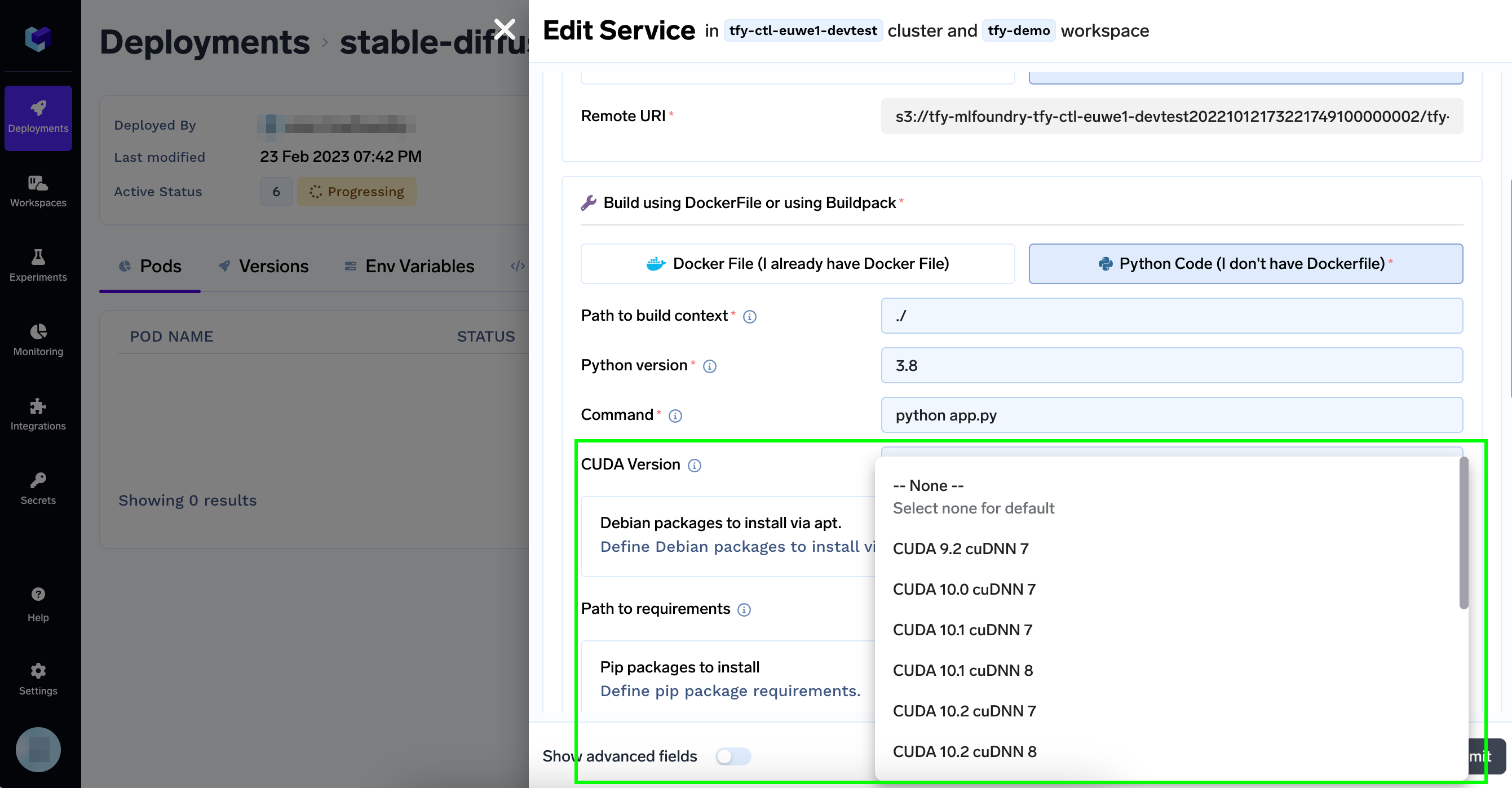
Select CUDA Version from the dropdown
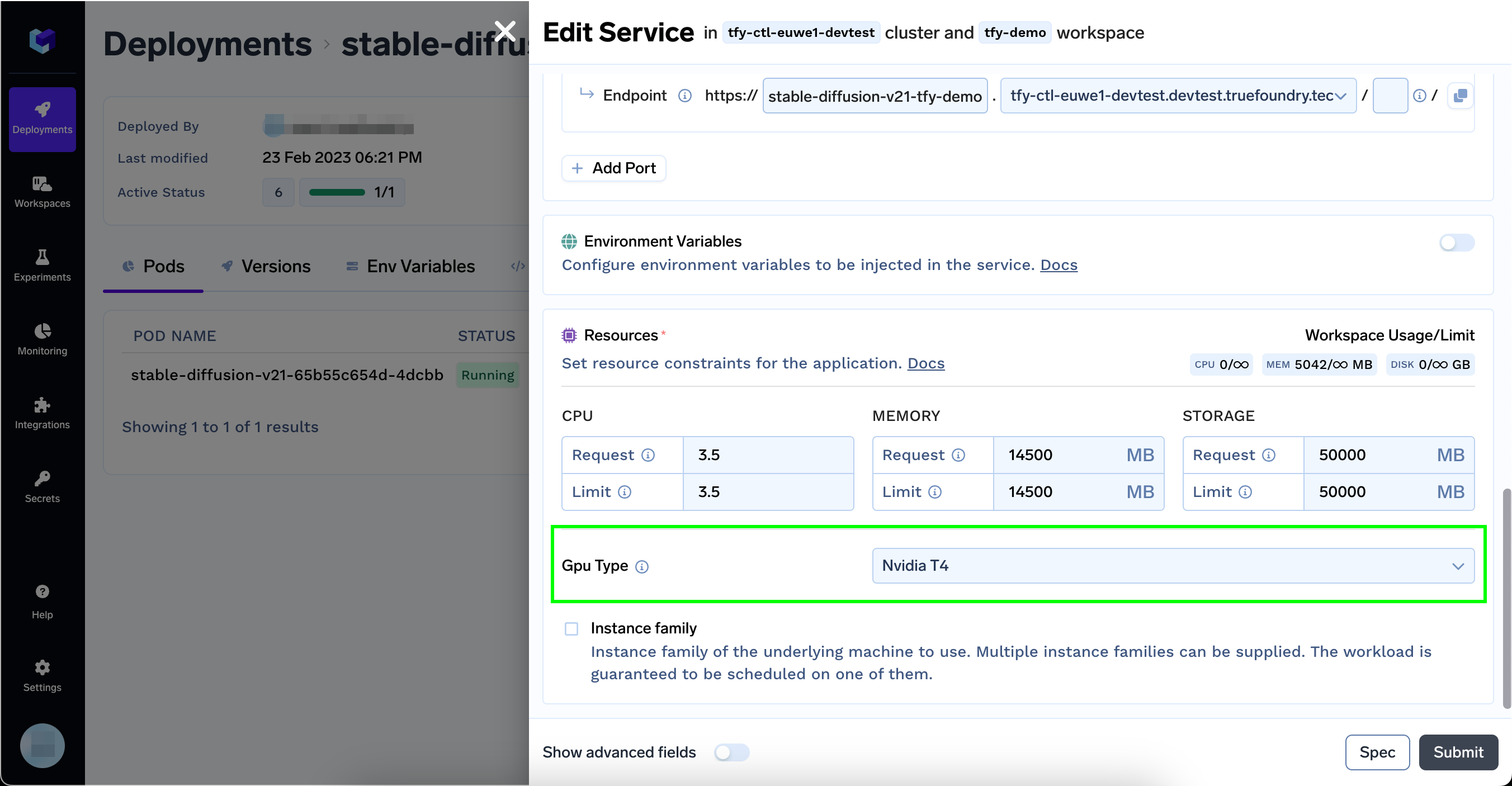
Select GPU Type from the dropdown
GPU Type Options
The GPU Type dropdown will only show GPUs that are available on instance families allowed on the selected workspace (by default all).
You can restrict the GPU instance types by editing the respective workspace.
Monitoring GPU Metrics
GPU Metrics are automatically captured and available in the Metrics
section of your Application
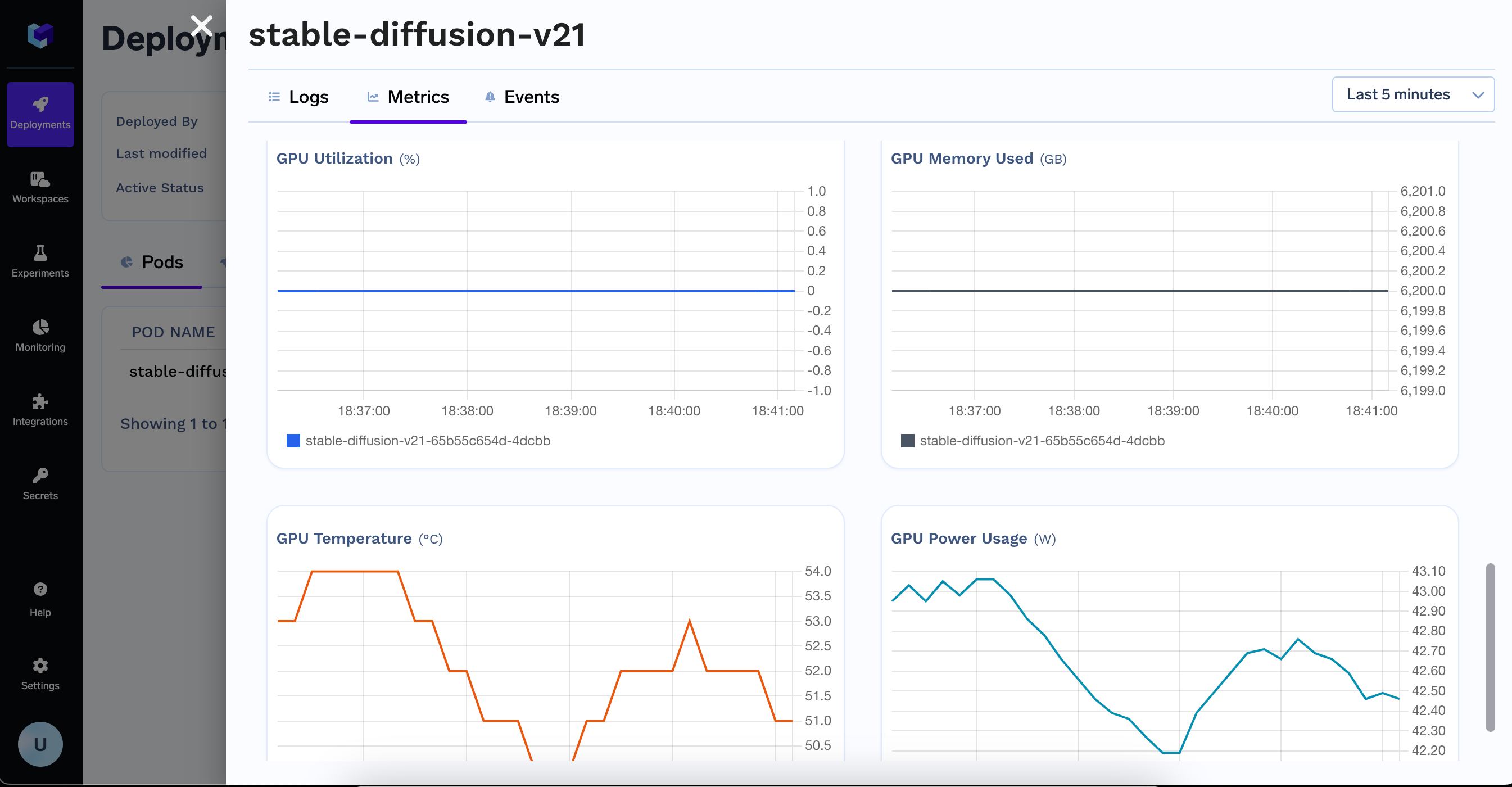
Updated 2 months ago